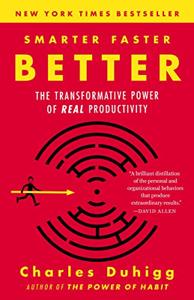
Want to learn the ideas in Smarter Faster Better better than ever? Read the world’s #1 book summary of Smarter Faster Better by Charles Duhigg here.
Read a brief 1-Page Summary or watch video summaries curated by our expert team. Note: this book guide is not affiliated with or endorsed by the publisher or author, and we always encourage you to purchase and read the full book.
Video Summaries of Smarter Faster Better
We’ve scoured the Internet for the very best videos on Smarter Faster Better, from high-quality videos summaries to interviews or commentary by Charles Duhigg.
1-Page Summary of Smarter Faster Better
Overview
Smarter Faster Better is a self-help book that describes ways to increase productivity. It uses scientific research and examples of how people can make decisions and assign their own significant meanings to them, which stimulates further action and motivation. Duhigg relates stories about teams being more productive when they are diverse, call on every team member to participate, and create a safe environment for team members.
The best way to set goals for a team is to have a stretch goal that meets the criteria of being specific, measurable, achievable, realistic and timely. A group can accomplish this with total effort. This goal should be meaningful and not distract from new goals when circumstances change in times of crisis; it’s important to visualize worst-case scenarios so you’re prepared if they happen.
Manufacturing and development methods, such as both Lean and Agile, empower people closest to the problem. These methods prevent resources from being tied up in bureaucracy. The Federal Bureau of Investigation’s Sentinel program is an example of this type of empowerment that resulted in productivity gains at a car factory.
When making decisions based on predictions, use models that take into consideration multiple probabilities and the potential profit or loss from those decisions. It is sometimes better to start off with a complex design first and then make it easier for people to understand later instead of starting out with an easy-to-understand model. This strategy is called “making things harder to understand at first” but in fact requires people to handle data in a less efficient way than normal. Some of today’s most creative minds are actually brokers who combine two previously established ideas in creative ways. Creativity also thrives under tension such as a deadline or a sudden change in environment.
Key Takeaways
When people believe they have the power to make choices and control outcomes, they’re motivated to do difficult things. The motivation comes from the meaning behind those choices.
The most productive teams are the ones that actively seek all members’ input, have clearly defined goals and rules of operation, trust one another to be honest with each other, and feel safe in proposing ideas to each other.
When we’re in a crisis, our focus becomes tunneled on the things that are wrong. This can lead us to overlook other important aspects of the situation. By having a mental model of what we should do during such situations and by visualizing the problem, we can prevent tunneling on the wrong things.
Goals should be specific, measurable, achievable and set a reasonable timeline. Decisions should be made by the people closest to the problem because they are more invested in the overall success of their team.
To predict the future, we should gather probabilities relevant to the situation and avoid focusing on successes. We should consider potential earnings and losses in our predictions as well as unknown factors.
Creative people combine existing ideas in different ways to create something new.
Creativity can be motivated by tension and change. Some people are forced to be creative because of a deadline, while other times the status quo needs to be disrupted before someone is inspired.
It’s possible to increase comprehension of data by forcing inefficient interactions with it. This can be achieved through disfluency, or making things harder for the brain to process. We can also encourage interaction with data and testing hypotheses about it.